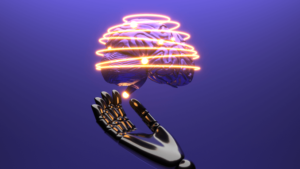
MLOPS
Simplify and amplify machine learning with strategies for growth and efficiency
Simplify and amplify machine learning with strategies for growth and efficiency
Having trouble implementing complex models? With containerization, automated workflows, and cloud platforms for smooth deployment, our MLOps expertise streamlines the process and frees you to concentrate on strategic projects.
Not gaining the anticipated gains on your machine learning investments? By finding areas for improvement and iterating for maximum ROI, we optimize model performance through A/B testing and constant monitoring using our MLOps strategy.
Are scalability and model performance a concern for you? We take advantage of MLOps techniques such as drift detection, autoscaling, and real-time monitoring to proactively handle problems and guarantee that models grow and adapt without any glitches.
Are ML projects taking too long to reach the market? Development and deployment cycles are accelerated by our optimized MLOps processes, such as automated workflows and infrastructure provisioning.
Not having sufficient internal knowledge to oversee ML operations? By managing infrastructure management, model deployment, and performance monitoring, we reduce the skill gap with our MLOps team.
Tight data compliance laws: a barrier? By using data governance tools, model interpretability strategies, and our in-depth knowledge of creating compliant ML pipelines, our MLOps solutions guarantee conformance.
To get better ROI, our experts align customer needs with your business goals.
01/08
Our group works together to identify the issue we’re trying to solve and how the ML model will help us achieve our goals.
02/08
In order to get ready for model training, we collect pertinent data, evaluate its quality, and obtain new insights.
03/08
To identify the most optimal solution, our data scientists create, train, and refine a range of models.
04/08
To select the top performer, we employ metrics to thoroughly assess candidate models.
05/08
For straightforward deployment and change tracking, we package the chosen model, code, and dependencies.
06/08
We automate training, testing, and data processing in our ML workflow to ensure effective updates.
07/08
Our team oversees the underlying infrastructure and deploys the model to a production environment.
08/08
To keep the model precise, we constantly monitor its performance, detect drift, and iterate.
We manage every step of your machine learning process, from gathering data to deployment and beyond, guaranteeing effective model creation and repeatability.
To improve your models, our team plans and carries out tests. We use innovative techniques to improve the performance of the model.
We put forth a strong framework to monitor every iteration of the model. Throughout the ML lifecycle, our team makes sure that the right governance is in place to preserve compliance and auditability.
For scalability and high availability, we smoothly integrate your models into production. For effective model serving, we leverage orchestration and containerization.
In order to identify performance problems, we regularly monitor deployed models. Our group optimizes models for long-term success and proactively discovers areas that need improvement.
To meet your demands, we choose and put into place the right MLOps infrastructure and technologies. The environment is managed and kept up to date by our staff to ensure scalability and seamless functioning.
For continuous training, deployment, and monitoring, we include machine learning workflows into your current CI/CD pipeline. Faster iteration and deployment cycles are made possible by this streamlining of the ML development process.
We enable your teams with tools they need to apply machine learning models successfully. To promote trust and openness, we offer resources and direction for deciphering and elucidating model behavior.
Through joint efforts, our team of technical and management specialists can expedite your projects.
Access to the top 2% of technical specialists for projects with shorter time-to-market that offer stability and scalability.
Reduce risk and maximise project optimization to ensure quality and on-time/budget delivery.
MLOps, or Machine Learning Operations, is a term used to describe the automation of the machine learning lifecycle, including development, deployment, and monitoring. It fills the void between data science and IT, ensuring ML models in production that operate effortlessly and predictably.
MLOps has a number of benefits, including enhanced cooperation, faster model deployment, more reliable models, data-driven decisions based on trust, and scalability for future expansion.
Even with a single ML model, MLOps is useful. It speeds up development, minimizes manual labor, and gets you ready for more machine learning projects.
Thanks to MLOps, data scientists can benefit from automated workflows, established data access, and additional time for innovation.
Choosing the appropriate tools, assembling a cooperative team, and integrating MLOps with the current infrastructure can all be challenging assignments.
Get exclusive insights, curated resources and expert guidance
Business Hub DHA Phase 8 Lahore
© 2024 | All rights reserved.